Will researcher diversity make AI algorithms more just?
And what does it mean for a dataset to be unrepresentative? Unrepresentative of whom?
Hey all, sorry for the late newsletter this week. I was feeling a little under the weather last week. But Iām back now, and ready to rumble. You can expect regular programming from now on šš½.
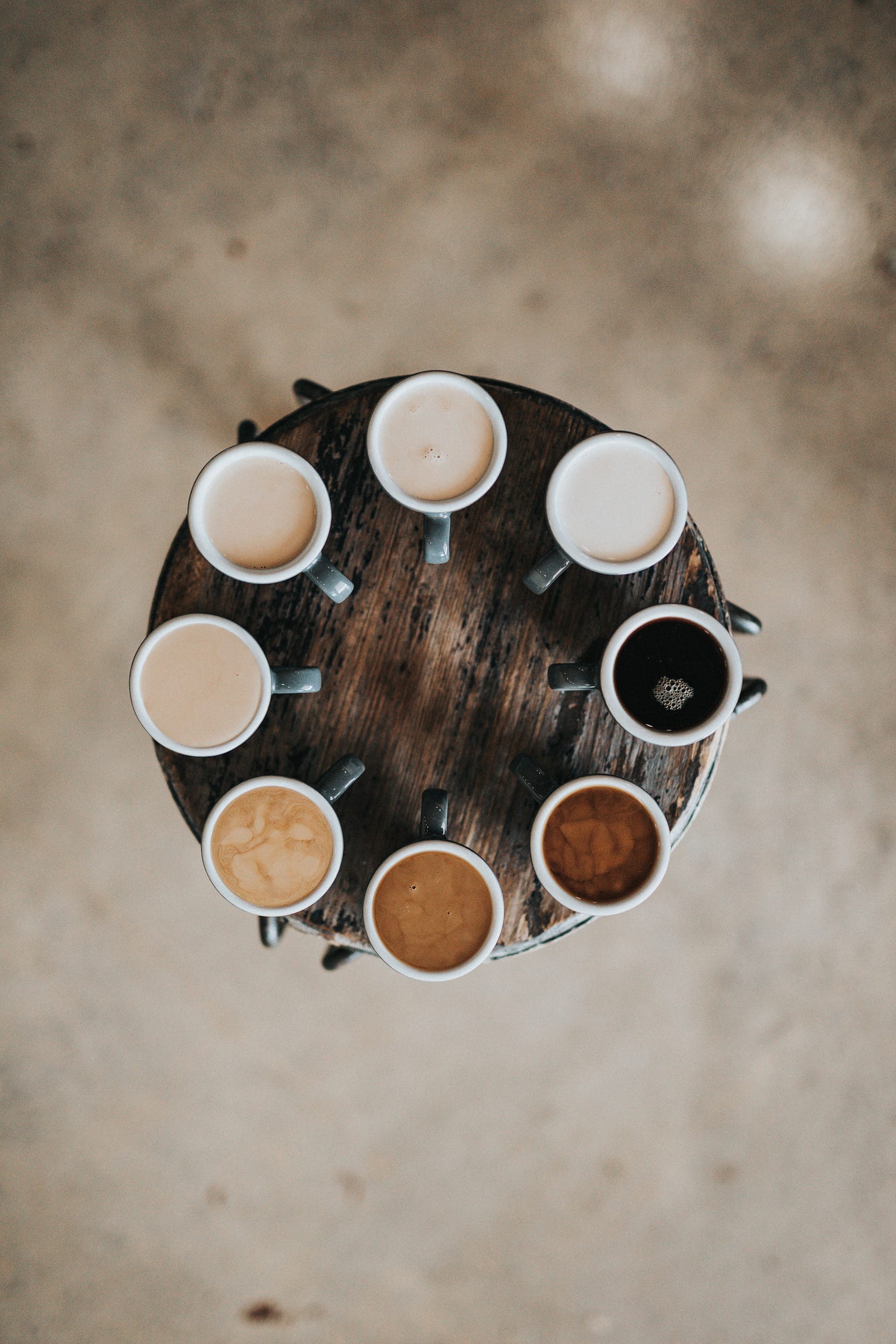
Today, Iām responding to an article published in the Doxa newsletter. The author writes about AI ethics from a perspective that isnāt common in the most popular tech media outlets. It is my hope that while we may agree or disagree on the tactics to a better AI ecosystem, we can inform and refine each otherās thinking over time.
In his article, he asks two questions that caught my attention.
Will researcher diversity make the AI algorithms more just?
What does it mean for a dataset to be unrepresentative? And unrepresentative of whom?
I have a simple answer to the first question. Yes. It absolutely will. I know this happens today, and will continue in the future. But you canāt hire a few minority AI researchers and expect your facial recognition technology to become unbiased. Itās deeper than the algorithms. Itās about power, budgets, and organizational will.
The second question sits squarely in the middle of the modern culture wars. On one side, the soldiers vehemently assert that the world today is imperfect and full of injustices and inequalities across all domains of life. It follows from this logic then, that one ought to create datasets that include and represent the experiences of the ignored and trampled upon. In the opposing corner, the defenders are pretty happy with the status quo. They might cede that inequality and injustices exist in some areas, but they do not support the massive upheaval of existing systems. This war is being fought on the battlegrounds of our colleges, in our media and literature, in the way we tell our history and in the apps we permit into our App Stores, This issue is far bigger than tech, and itās not going away anytime soon.
Letās dig in.
Will researcher diversity make the AI algorithms more just?
Jon argues:
āļøTLDR (too long, didnāt read)
Incentives are important, but they do not tell the whole story. I mean, BP is incentivized to keep its rigs safe. But the Deepwater Horizon rig blew up killing 11 people and ripped a $65 billion hole in BPās wallet. Blindspots are real. You canāt see what you canāt see. Power corrupts and people cut corners. Best intentions are often woefully insufficient. Good, sensible ideas like investing in safe rigs and building fair AI often fail because of a lack of organizational will.
Imagine the engineers working on the first Snapchat filter. Theyāre testing the very first prototype on themselves. You can easily assume that a diverse group of engineers would notice if the duck face filter didnāt work well on non-white skin. A diverse group would likely have different ideas of whatās considered acceptable and whatās not. Sure, there are small but growing numbers of non-white AI researchers at big tech companies today. But show me the budget and Iāll tell you how much these companies truly care.
Which number do you think is higher:
How much an AI company spends on company snacks?
How much they spend specifically trying to make their algorithms accessible and fair?
I donāt presume to know the answer but I wonāt be surprised if the numbers are closer than further apart.
šTSWM (too short, want more)
āļø Product failures should be called they are
I reject the framing that changing commercial AI models in support of greater diversity are āsocial justice changesā. If these companies want to be global, and they want to serve diverse groups of people, their AI models need to cater to the demographics of the world. And if they donāt, their products have failed. Itās as simple as that. Itās like Amazon checkout but it only works for 53 percent of the population. Or a kettle that only works on Tuesdays. That would be ridiculous, no?
So when facial recognition tech fails more on black people - up to ten times more than on white people - that product has fundamentally failed. Letās call that what it is. Itās not a car with an intermittent flashing ācheck engineā light. Itās a car with an exploded engine. And what do you do when a product fails? Go back to the drawing board to reassess the requirements.
šÆ Mathematical definitions of fairness
Thereās active research in algorithmic fairness and algorithmic justice where people are exploring different approaches to make sure algorithms are fairer for all. The first step is identifying that harm could in fact be caused. To make things concrete, researchers have created strict definitions of what we mean when we say āfairā. These definitions vary quite a bit. In fact, one researcher found that there are up to 21 mathematical definitions of fairness. In the book, the Ethical Algorithm, the authors describe how optimizing for one kind of fairness means you get less accuracy or less of another type of fairness. So itās not immediately obvious that thereās a simple āright solutionā.
Given a curve that describes algorithmic accuracy and different kinds of fairness, society will have to choose which point on the curve we want to sit on. That location will vary with application. For some applications, itās far worse to have a false positive than a false negative. So we might agree, that all protected groups should have equal rates of false positives. In other situations, we might choose otherwise. Like many ethical dilemmas, there is no blanket, context-independent prescription that would make sense in all cases. Thereās a similar conversation going on in the biotech world with respect to CRISPR. When (if ever) is it acceptable to edit the germline of a baby? Say a baby has a terrible genetic disease, is it worth trying to cure the baby? Knowing that CRISPR is notorious for off-target edits (basically, genetic mistakes) and germline editing means that the babiesā future lineage will carry these mistakes in perpetuity. Is it worth the attempt? Maybe in the future when the precision is better but Iād argue weāre not there yet.
š Experiences > ābrain trustā
The focus on the āAI brain trustā here is a bit misplaced. Biases that creep into products come from many different sources. Itās not just the training data. The algorithm design might be at fault, or the training data could contain historical biases. Or it could be that the product is just a bad idea that will disproportionately lead to more negative outcomes for some groups over others. The work to fix these biases isnāt on anyone single team.
In tech companies today, we already see that underrepresented employees end up doing the unpaid work of highlighting product failures that happen to minorities. Whether itās Google Photos labeling black people as gorillas (yes, that really happened), or itās searching ācornrowsā (an African/African-American hairstyle) and only seeing images of white people. People from diverse backgrounds will always be able to highlight these issues far better than homogenous groups. That said, the expectation should never be that minorities have to shoulder the extra weight. Product leaders need to empower their organizations to fix these product failures, or nothing will change.
What does it mean for a dataset to be unrepresentative? And unrepresentative of whom?
Jon argues:
The AI ethics activists don't like that these large AIs are trained on data from the normie world of microaggressions, gendered language, and implicit biases ā they're trying to destroy that world, so they'd not see it perpetuated by a globe-spanning, energy-sucking artificial intelligence.
To paint a more concrete picture of what this might look like: the ethics people want to be able to do for the language used by consumer products like Alexa and the Google search box what activist orgs are already doing to great effect for the media, i.e., circulate a guide for what to say and what not to say (likeĀ this, orĀ this).
āļøTLDR (too long, didnāt read)
There are complex sociological, political, and moral layers to this question. The part Iām focusing on is this - dear reader, what do you consider the role of technology to be? Is it to reflect the world as it is today in its beauty and imperfections? Or is technology a wand with which we can begin to rid the world of its ills in order to create a more just, more inclusive world?
Imagine youāre collecting data about the history of the US. Some texts will over-index on the Christopher Columbus savior narrative. Some might even suggest indigenous people ājust sorta conveniently disappearedā. Meanwhile, texts written and championed by other voices will tell a rather different story. One that is brutal, painful, and is not recommended bedtime reading for children.
Which story do you take? Do you take both? Can you reconcile such opposing data?
If you over-index on the story told by the colonizer, you will miss a lot of useful information. Likewise, if you over-index on the indigenous stories, youād probably miss a lot too. Iād argue you take both, but unfortunately, weāre getting to a place in the US where weāre struggling to agree on the events of the previous day. So as we feed our machines with data and design algorithms to glean inferences from them, we have to proceed with caution.
šTSWM (too short, want more)
š¤Siri vs your mother
I think technology should reflect the world as it is while striving to be inclusive and compassionate. To the extent that itās possible, Siri should not be a micro-aggressor. That said, I can understand why Siri might, for example, use a male pronoun when referring to a lorry driver. If Siri is trained on US employment data, it will learn that most lorry drivers are male. This is an undeniable fact. You can argue whether the gender of a lorry driver is even important but bear with me. Siri should participate in speech like a decent human would. But whatās ādecentā in San Francisco circles is very different to whatās decent everywhere else.
If your mother assumed a lorry driver was male. Would that offend or bother you? Are you holding technology to a standard you wouldnāt hold your dearest? Maybe you're holding everything to that standard because you think all gender-based assumptions are wrong and dated. But do you think word bans and circulated guidelines can be effective? Especially when you consider that these products are global. Tell me in the comments!
šInternet data is not evenly representative
Many large AI models are trained on Internet texts. These texts are not distributed in a way thatās inclusive of everyone. Even though we might think of the Internet as widely open and accessible - there are groups online that find it harder to have communities online. These include older people, people with disabilities, and people who are at the intersection of multiple marginalized characteristics. Consequently, there are fewer sites of these groups. So thereās less āInternet dataā of these groups. And itās entirely feasible that the data from these groups is overlooked or unprioritized when designing and building AI products.
āØTalk to Tobi
These questions were somewhat spicy. What do you think? Tell me in the comments!
If you over-index on the story told by the colonizer, you will miss a lot of useful information.
Can I get this on a shirt?
Great article! I really enjoyed how you unpacked both sides on this supercharged topic, in a balanced and informative way (something rare these days). One of the best newsletters out there, looking forward to the next one!